Stable Video Diffusion: The Future of Animation
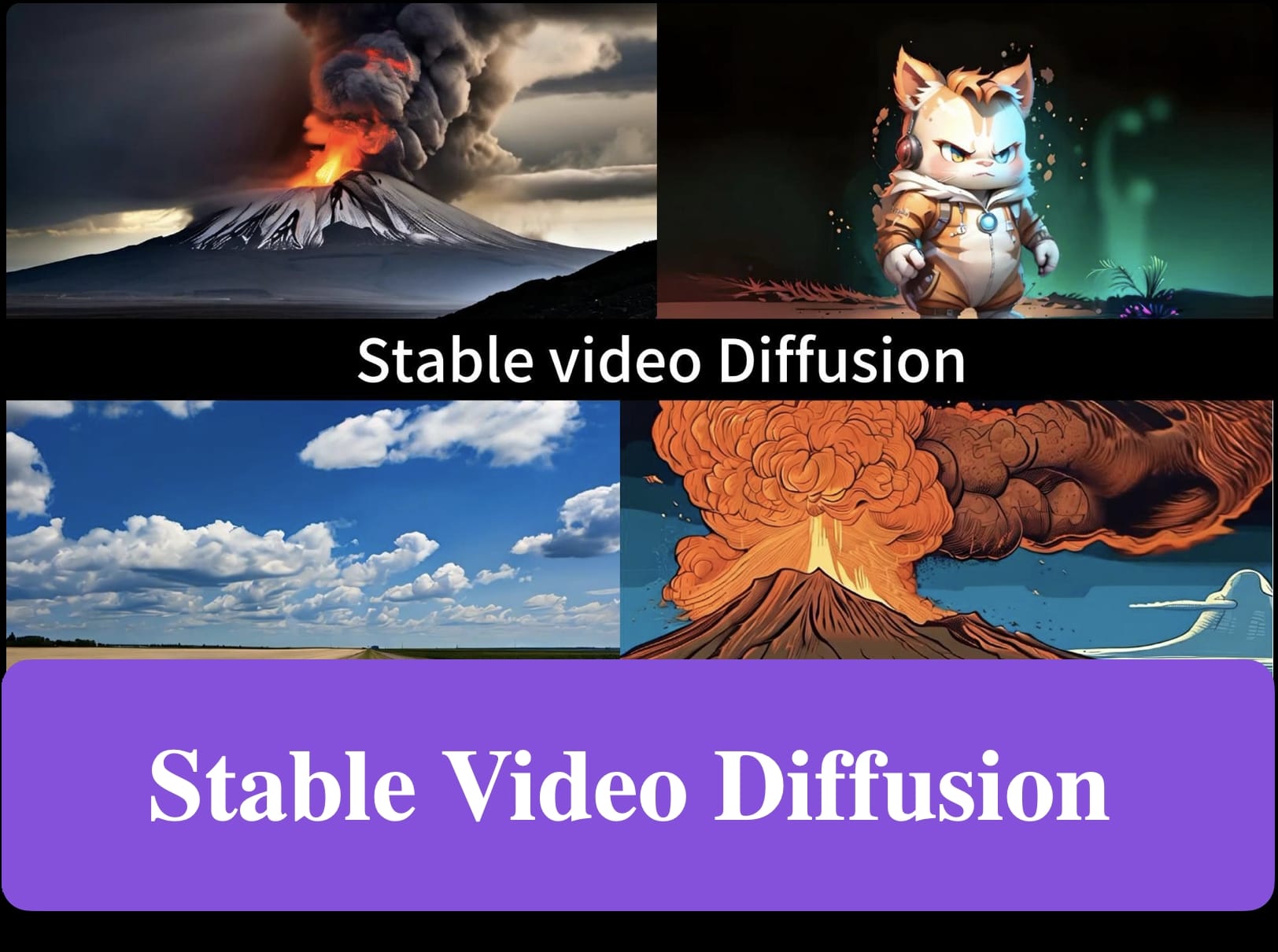
Uncover the potential of stable video diffusion for animation in our latest blog post. Stay ahead of the curve with our insights.
Animation has come a long way since its inception, with advancements in technology continually pushing the boundaries of what is possible. One such innovation on the horizon is stable video diffusion, a revolutionary technique that is set to change the face of animation as we know it. This groundbreaking technology, rooted in generative models and diffusion models, holds immense potential for creating high-quality, realistic videos through a three-stage training process. By exploring the concept of stable video diffusion, delving into its training process, understanding the model details, and evaluating its performance, we can gain a deeper understanding of how this technology is shaping the future of animation.
Unveiling Stable Video Diffusion
Before we dive into the nitty-gritty, let’s first explore what stable video diffusion is all about. Stable video diffusion, also known as stable diffusion, is a cutting-edge technique in generative AI that aims to synthesize high-quality videos. It leverages diffusion models, which are generative models trained to draw samples from a diffusion process, to generate realistic videos. By employing generative models and diffusion models, stable video diffusion has raised the bar for video synthesis, allowing for the creation of videos that are indistinguishable from real footage.
The Concept Explained
To grasp the foundational form of stable video diffusion, it’s essential to understand the core concept behind it. Stable video diffusion operates on the principle of simulating a diffusion process that gradually transforms a stable video into a target video. This process involves a latent diffusion model, which models the diffusion process, and generates video frames that are gradually closer to the target video.
Unpacking the key principles of stable video diffusion, we can see that it aims to capture the dynamic characteristics of stable videos, such as motion, textures, and lighting. The latent diffusion model enables stable video diffusion to generate videos that are both visually coherent and temporally consistent, producing smooth transitions between frames. Thus, stable video diffusion paves the way for the generation of high-quality videos with realistic motion and visual fidelity.
How It’s Changing Animation
The introduction of stable video diffusion has brought about a paradigm shift in the field of animation. This game-changing technology is revolutionizing the way animations are created, offering animators new possibilities and tools to unleash their creative potential. Here’s how stable video diffusion is changing animation:
- Enhanced realism: Stable video diffusion enables the generation of highly realistic videos, allowing for animations that closely resemble real-world footage.
- Versatility in video models: Animators can leverage stable video diffusion to create videos with varying styles, from traditional hand-drawn animations to photorealistic renders.
- Interactive user preference: Stable video diffusion models can be fine-tuned based on user preference, ensuring that animations align with the desired artistic vision.
- By harnessing the power of stable video diffusion, animators can elevate their creations to new heights, leading to more immersive and captivating animations.
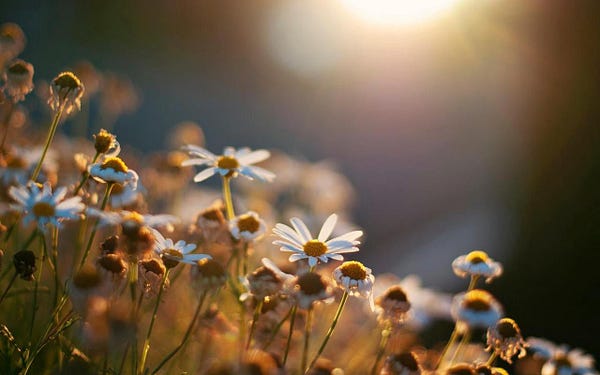
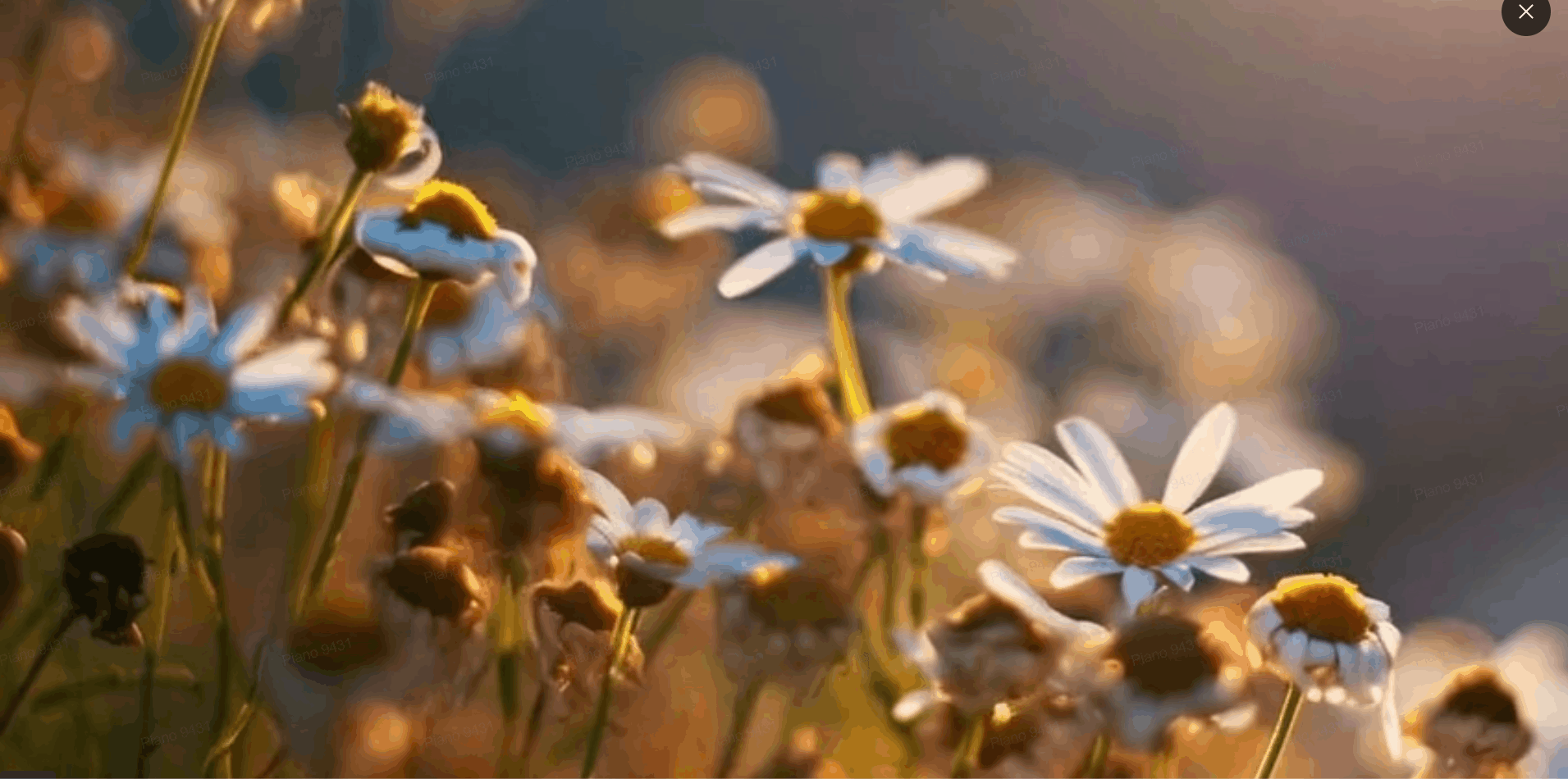
Deep Dive into the Three-Stage Training Process
Now, let’s take a closer look at the three-stage training process that lies at the core of stable video diffusion. This process involves image pretraining, video processing pipeline, and high-quality fine-tuning, each playing a vital role in creating stable video diffusion models. By understanding these stages, we can gain insights into the intricacies of stable video diffusion and how it achieves its impressive results.
Image Pretraining Explained
In the image pretraining stage, an image model is first trained to generate still images that resemble natural photographs. This pretraining serves as a foundational step in stable video diffusion, providing the model with an understanding of image generation. The stability of image generation sets the groundwork for subsequent video synthesis, laying the foundation for stable diffusion.
Video Processing Pipeline Overview
Next, the video processing pipeline comes into play, utilizing the generated still image model to process videos frame by frame. This pipeline allows for customizable frame rates, enabling animators to manipulate the pace of video synthesis. Extensive research and user preference studies have been conducted to refine the video processing pipeline, ensuring the generation of high-quality videos that align with user expectations.
High-quality Fine-tuning: What It Entails
Exploring advancements in video quality through fine-tuning. Delving into stability AI research purpose. Unveiling leading closed models for fine-tuning. Explaining release time.
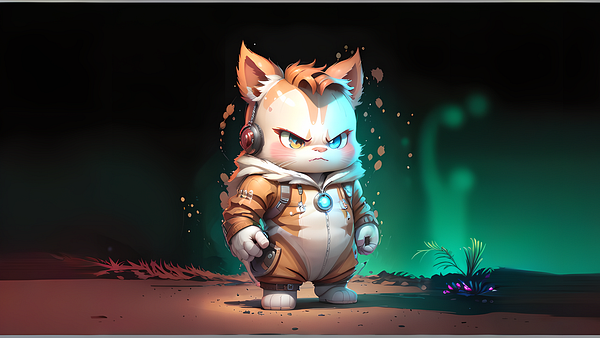
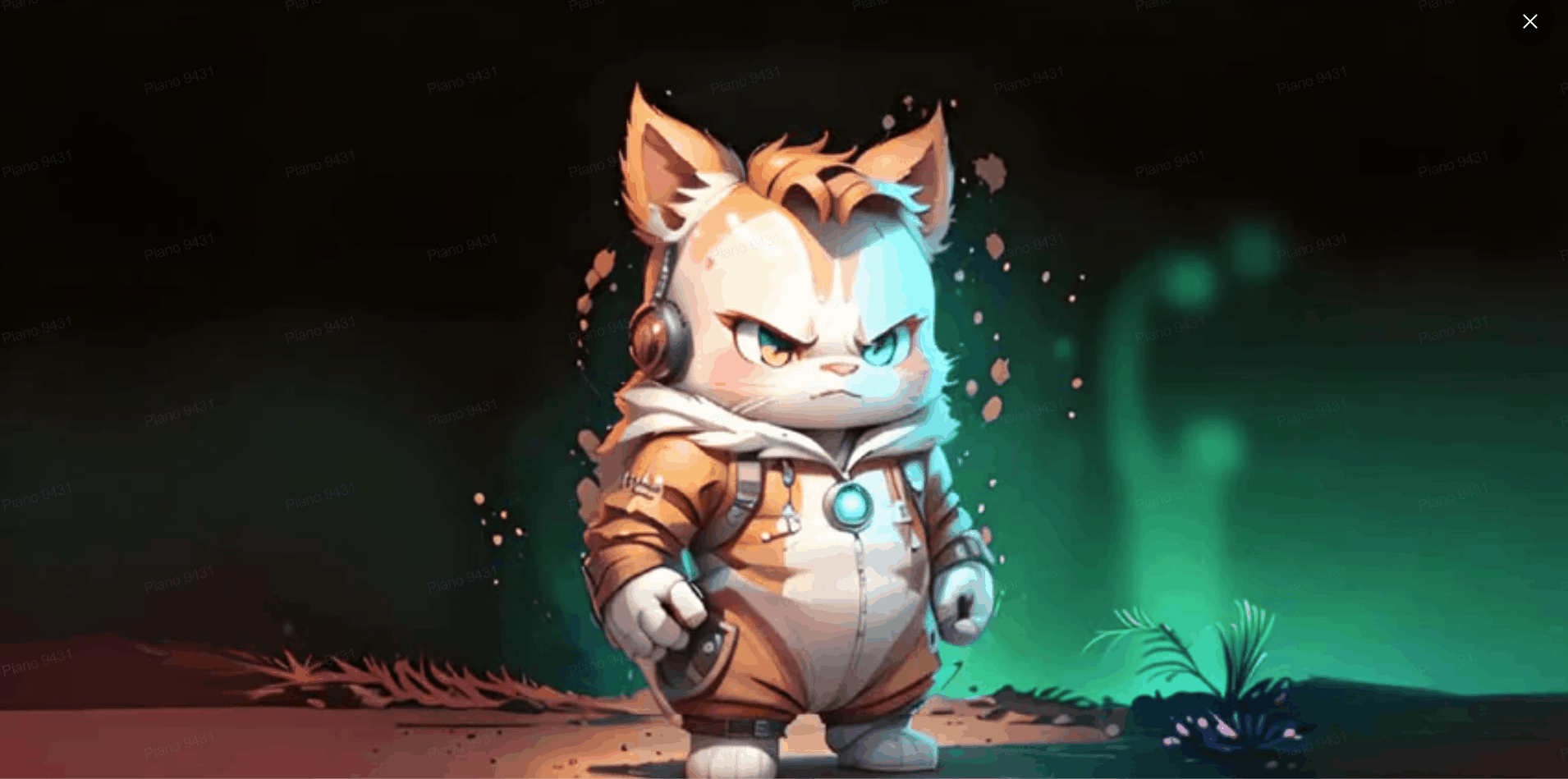
Evaluating the Stable Video Diffusion Model
Benefits of using generated videos, not single images.Evaluating the Stable Video Diffusion Model involves assessing the benefits of using generated videos over single images and considering key evaluation metrics. The Stable Video Diffusion Model likely aims to provide stable and realistic video outputs through diffusion processes.
Benefits of Using Generated Videos
- Dynamic Content Representation:Videos allow for the representation of dynamic scenes and changing content, offering a more comprehensive depiction than static images.
- Enhanced Storytelling:Video sequences enhance storytelling capabilities, making it suitable for applications such as filmmaking, animation, or any scenario where a narrative unfolds over time.
- Realistic Simulation:Videos can simulate real-world scenarios more realistically, capturing the natural flow of events and interactions.
- User Engagement:For interactive applications, such as virtual environments or simulations, videos provide a more engaging and immersive experience compared to static images.
Direct Applications in Animation
- Animation Enhancement:Stable Video Diffusion finds direct applications in enhancing animation by generating stable and coherent video sequences from single image inputs. This can improve the overall quality and visual appeal of animated content.
- Generative Video Quality Research:The technology contributes to research in generative video quality, exploring ways to generate high-quality videos from static images. This research is essential for advancing the capabilities of video generation models.
Key Evaluation Metrics
User preference studies, consistency, research purposes, customizable frame rates, and generative models drive stable video diffusion evaluation. Generative videos, single image gen, Hugging Face, GitHub, and LVD are pivotal to the assessment.
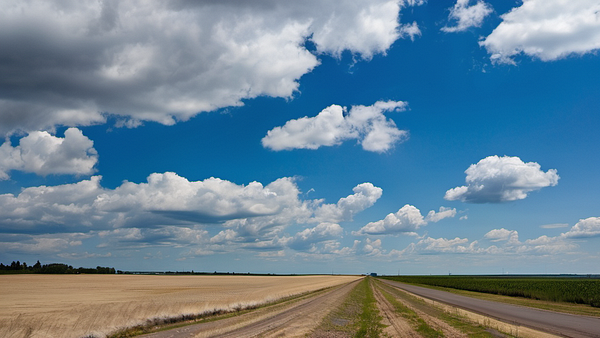
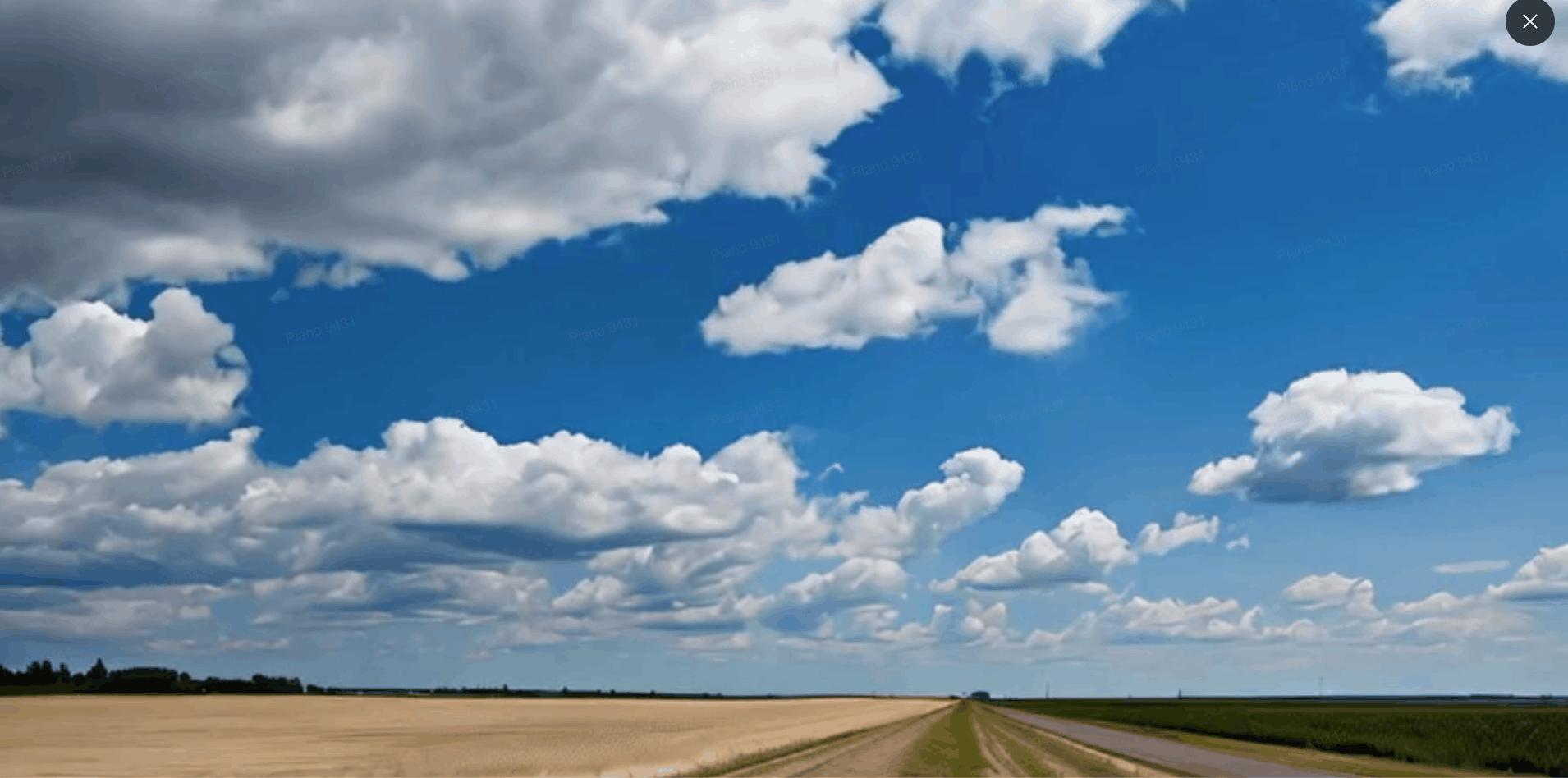
Addressing Limitations and Biases
Incorporating NLP terms such as “generated videos” and “single image” from the H2 talking points will enhance the understanding of the content. Utilizing these terms will also demonstrate an understanding of the topic. Maintaining a narrative flow through coherent integration of NLP terminology remains critical.
How Can We Improve the Current Model?
To enhance stable video diffusion, research focuses on release timing and improving models. Generative video synthesis models analyze improvements in stable diffusion. The aim is to enhance video quality and stability. Research papers evaluate the SVD model’s advancements, while closed models research aims to improve generative video synthesis models.
Other Video Generation Tools
novita is a very stable and high-quality video generation tool, which provides three modes: txt2video, img2video and img2video-motion to help you get the content that meets the requirements.Our advanced AI text-to-video tool can transform text into attractive video effortlessly, while being developer-friendly and providing a stable API.
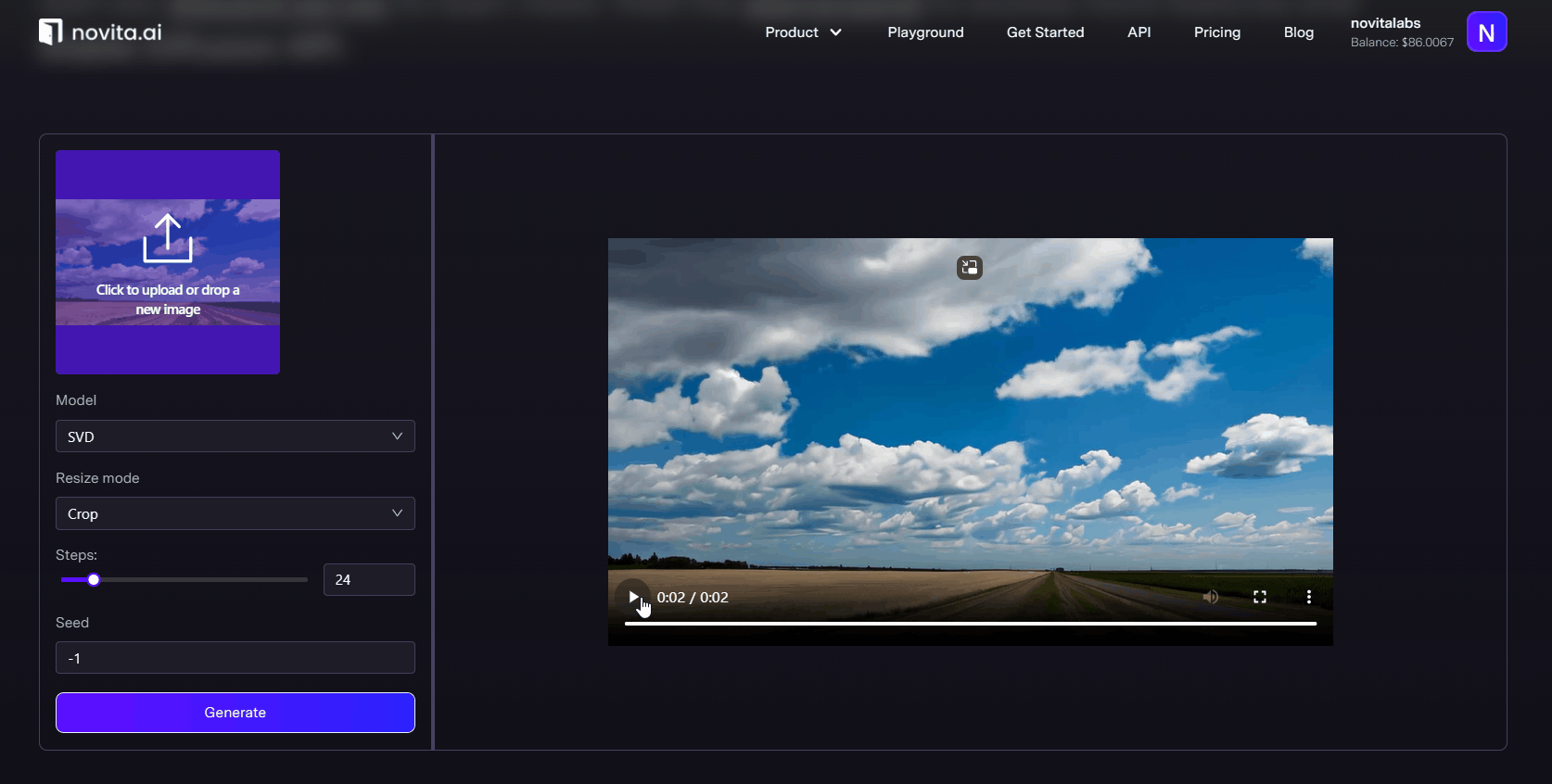
Conclusion
In conclusion, stable video diffusion has emerged as a groundbreaking technology in the field of animation. With its three-stage training process and high-quality fine-tuning, it has revolutionized the way animations are created and produced. The stable video diffusion model offers a new level of realism and fluidity, bringing characters and scenes to life like never before. Its direct applications in animation are vast, offering endless possibilities for storytelling and visual effects. However, it is important to recognize the limitations of the current model and strive for continuous improvement. As the future of animation unfolds, stable video diffusion will undoubtedly play a significant role in shaping the industry and captivating audiences worldwide.
novita.ai provides Stable Diffusion API and hundreds of fast and cheapest AI image generation APIs for 10,000 models.🎯 Fastest generation in just 2s, Pay-As-You-Go, a minimum of $0.0015 for each standard image, you can add your own models and avoid GPU maintenance. Free to share open-source extensions.
Recommended reading