5 Best GPUs for AI 2024: Your Ultimate Guide
GPUs now play a crucial role in powering AI programs. We will list top 5 GPU include RTX4090 with key features and function comparison.
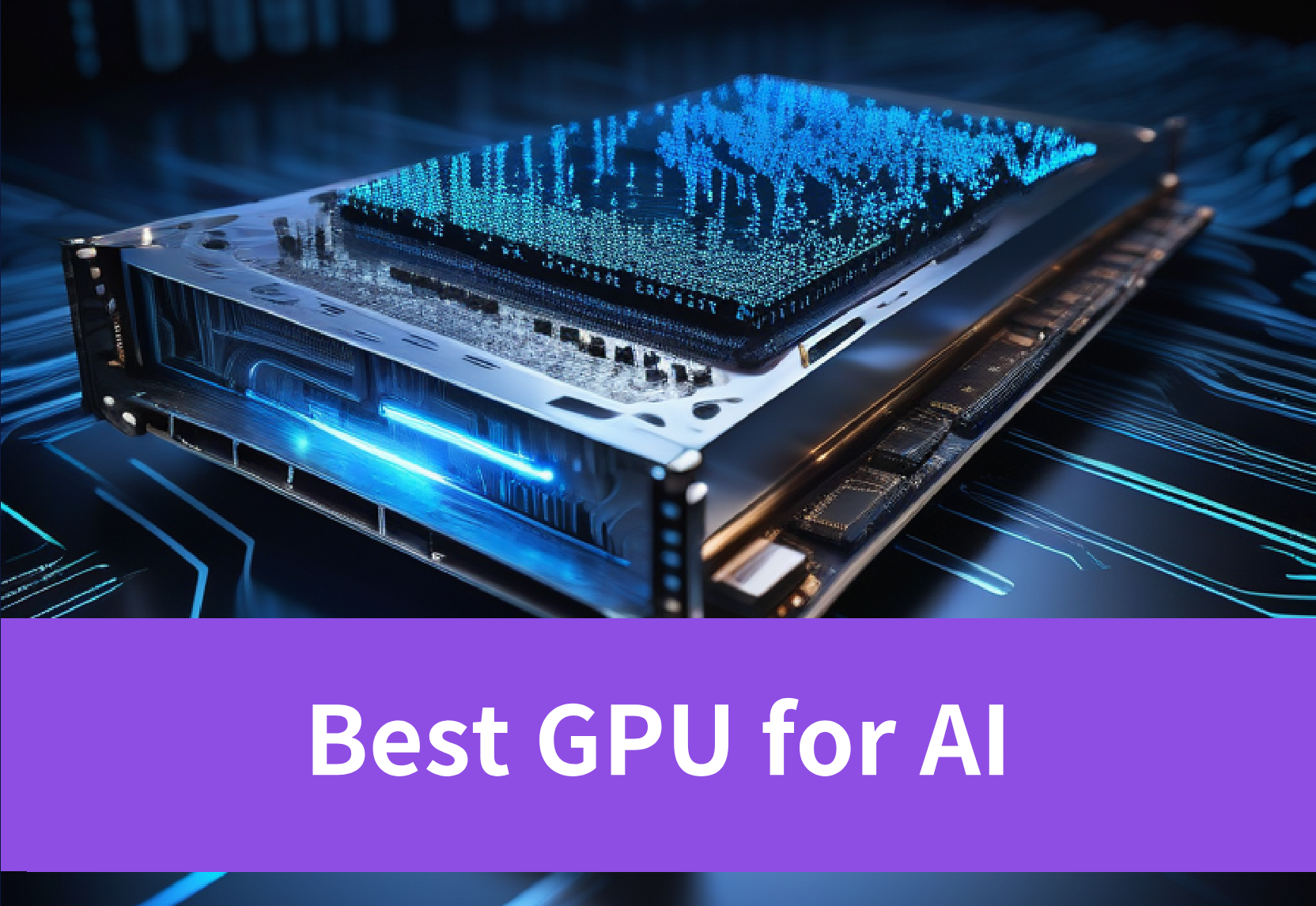
Key Highlights
- GPUs, originally designed for video game graphics, now play a crucial role in powering AI programs.
- We will talk about three top-notch GPUs that come highly recommended for working on AI projects, such as NVIDIA A100, NVIDIA RTX A6000, NVIDIA RTX 4090, NVIDIA RTX 3090, NVIDIA V100.
- Key Features to Consider When Choosing a GPU for AI.
- Try rent GPU in Novita AI GPU Instance to experience different GPU conveniently and reduce your cost and enhance your workflow in the GPU cloud.
Introduction
GPUs, originally designed for video game graphics, now play a crucial role in powering AI programs. Unlike CPUs, GPUs excel at handling multiple tasks simultaneously, enabling faster learning and decision-making for AIs.
In our deep dive into GPU selection for successful AI and deep learning projects, we’ll explore the top-performing GPUs. Discover what makes them stand out, their speed capabilities, and key differentiators. Whether you’re in data science, researching new technologies, or passionate about artificial intelligence, this guide will emphasize the importance of selecting the right GPU and provide essential criteria for your decision-making process.
Top 5 GPUs for AI in the Current Market
Right now, the market is full of GPUs that are perfect for AI tasks. Let’s talk about three top-notch GPUs that come highly recommended for working on AI projects:
NVIDIA A100: The AI Research Standard
The NVIDIA A100 is a top choice for AI research, thanks to its Ampere architecture and advanced Tensor Core technology. It excels in deep learning tasks and AI training, providing high memory bandwidth and superior processing power. Ideal for deep learning research and large language model development, the A100 meets the demanding needs of modern AI applications.
NVIDIA RTX A6000: Versatility for Professionals
The NVIDIA RTX A6000 is versatile, catering to various AI professional needs. With excellent GPU memory and bandwidth, it handles deep learning, computer vision, and language model projects efficiently. Its Tensor Cores enhance AI acceleration, making it a great choice for demanding AI workloads, balancing high performance with robust handling capabilities.
NVIDIA RTX 4090: The Cutting-Edge GPU for AI
The NVIDIA RTX 4090 represents the pinnacle of GPU technology for AI applications. Whether training deep learning models or processing vast datasets, the RTX 4090 ensures unparalleled speed and efficiency, making it the ultimate choice for AI professionals seeking the best in GPU technology.
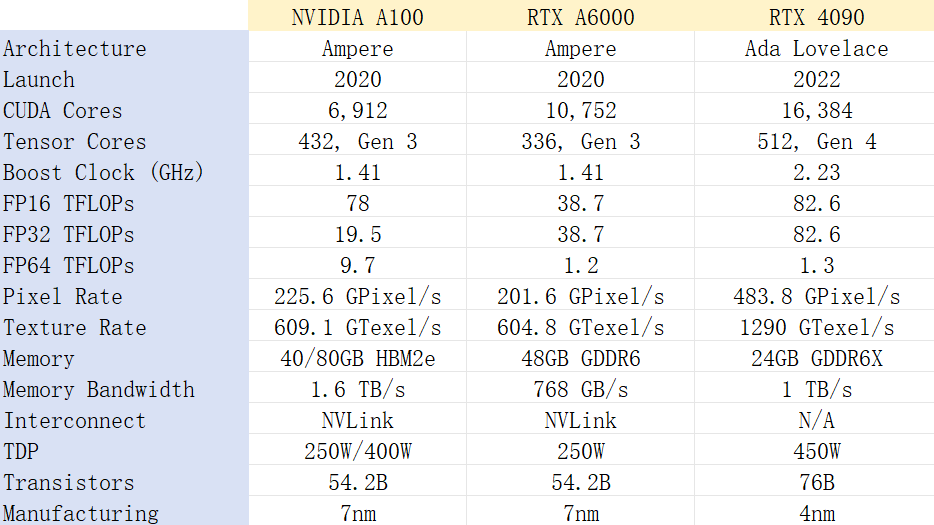
NVIDIA V100
The NVIDIA V100 is an excellent GPU for deep learning. It is designed specifically for high-performance computing and AI workloads, making it well-suited for deep learning tasks.
NVIDIA RTX 3090: (Previous generation)
The RTX 3090 has 10,496 CUDA cores, providing exceptional parallel processing capabilities, ideal for AI working. And it has a huge 24 GB of G6X VRAM, giving you lots of memory for games that need a lot of graphics power or playing at high resolutions. Its Tensor Cores enhance AI acceleration, making it a great choice for demanding AI workloads, balancing high performance with robust handling capabilities.
Key Features to Consider When Choosing a GPU for AI
When picking out a GPU for AI tasks, it’s important to look at several crucial aspects:
1. Understanding CUDA Cores and Stream Processors
CUDA cores, also known as stream processors, are vital for modern graphics cards, especially in AI tasks. The number of CUDA cores in a GPU affects its speed and power, enabling faster training and smarter AI models.
These cores efficiently handle multiple tasks simultaneously, breaking down big computing chores into smaller bits, thus accelerating data processing. When selecting a GPU for AI projects, the number of CUDA cores is crucial for better performance and increased productivity.
2. Importance of Memory Capacity and Bandwidth
Memory capacity and bandwidth are critical when choosing a GPU for AI tasks. Ample memory allows the GPU to handle large datasets and complex models without running out of space. Faster memory enables quicker data transfer, reducing wait times during calculations, which is particularly beneficial for deep learning projects. For efficient AI model training, a GPU with substantial memory and high-speed bandwidth is essential for smoother and quicker processing.
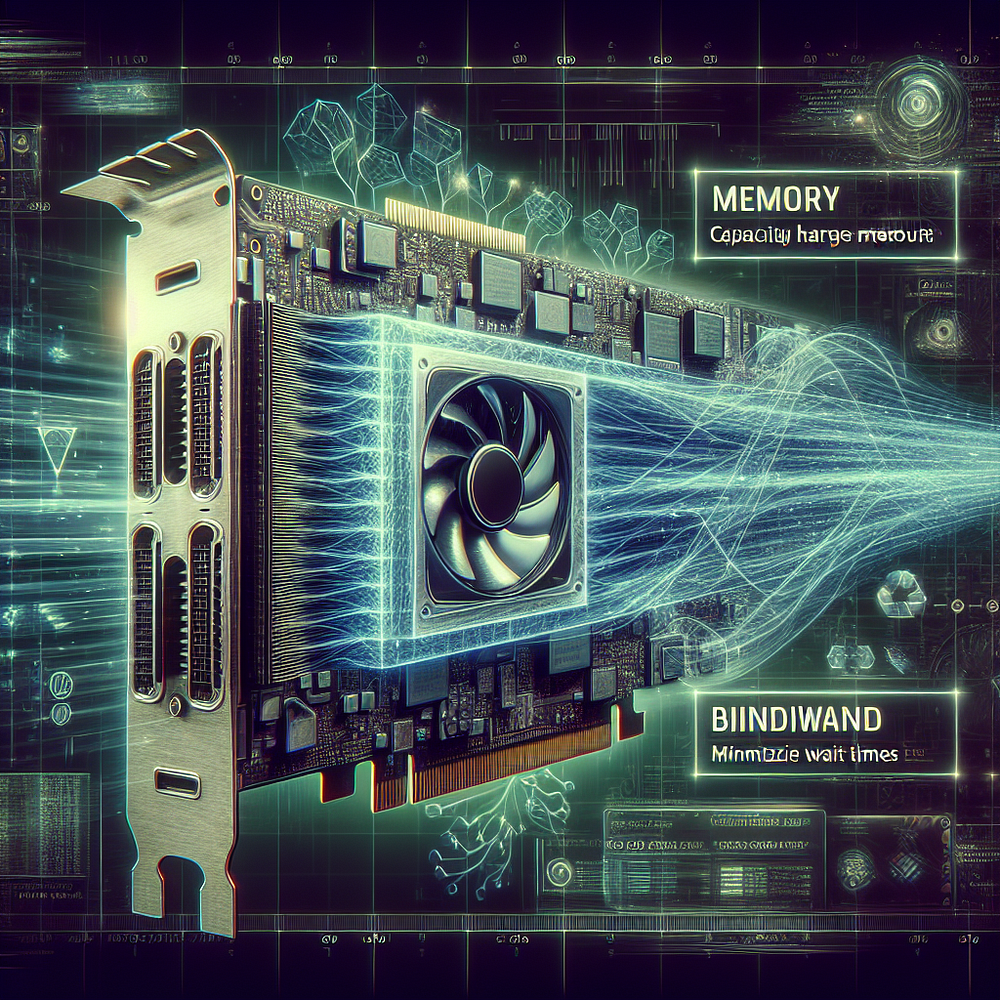
3. Tensor Cores and Their Role in AI Acceleration
NVIDIA GPUs feature Tensor Cores, specialized for speeding up AI tasks, especially matrix multiplication in deep learning algorithms. Tensor Cores enhance computing power, making training and inference faster by mixing different types of calculations. This efficiency allows for quick processing without excessive memory use or detail loss. For optimal AI performance, selecting a GPU with Tensor Cores ensures faster and smoother operations in machine learning and deep learning projects.
4. Budget Considerations
When on a budget, finding a GPU that balances performance and cost is key. Look for models that offer a good number of CUDA cores, sufficient memory, and decent bandwidth without the high price tag of top-tier options. Mid-range GPUs often provide excellent performance for many AI tasks without the hefty cost. While they may lack Tensor Cores, they can still handle most machine learning and deep learning tasks effectively, making them a great choice for budget-conscious AI enthusiasts.
Optimizing Your AI Projects with the Right GPU Configuration
When you’re working on AI projects, it’s really important to think about the GPU setup. You’ve got to look at a few things to make sure everything runs smoothly and efficiently.
Balancing GPU Power with System Requirements
Ensuring your GPU power aligns with system capabilities is crucial for AI projects. Consider the GPU’s power consumption and check if your system supports it. High-power GPUs might need extra cooling or a larger power supply. Balancing GPU strength with system requirements ensures efficient and harmonious operation.
Strategies for Multi-GPU Setups in AI Research
Using multiple GPUs can significantly enhance AI research by speeding up model training and data processing. Connecting GPUs with technologies like NVIDIA’s NVLink improves communication and memory sharing. Optimizing task distribution across GPUs maximizes performance. This multi-GPU approach accelerates AI research and yields faster results for large models.
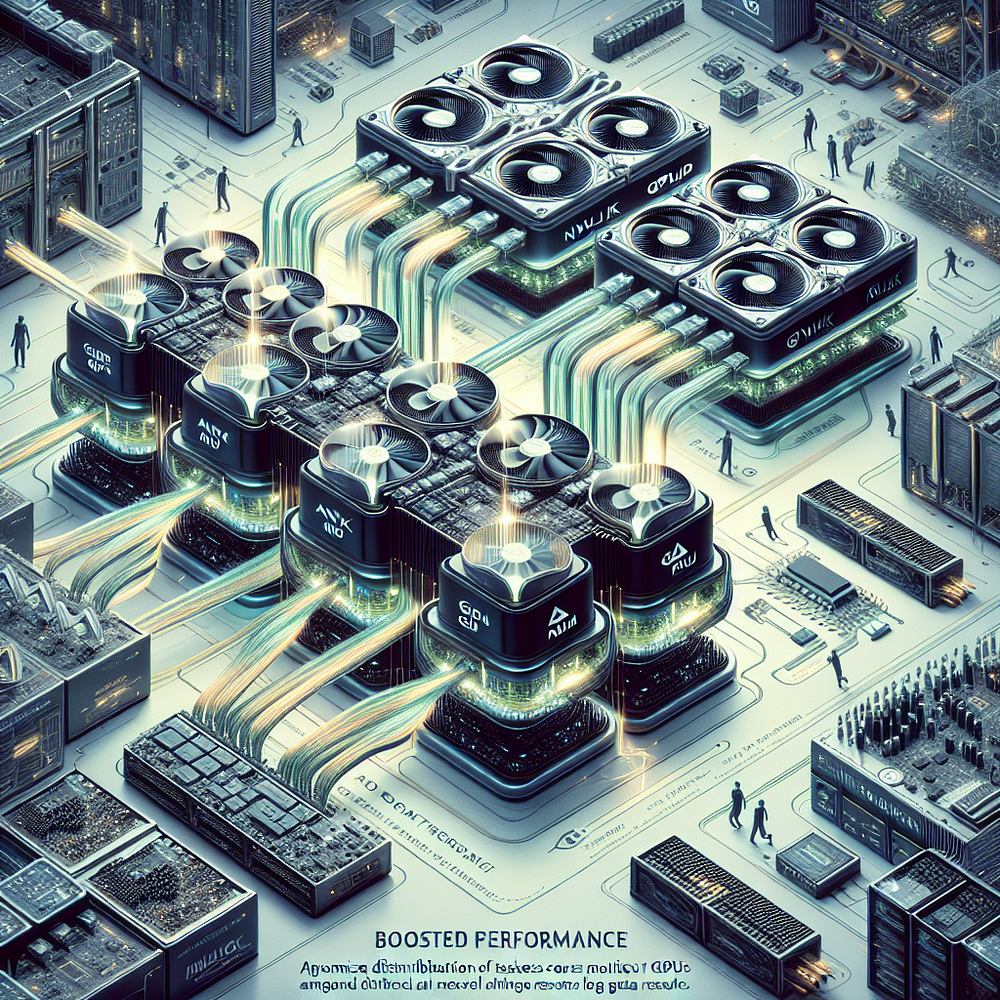
Experience GPU Cloud to enhance your AI workflow
With the rapid development of artificial intelligence and deep learning, the demand for GPU cloud services continues to grow. More enterprises and research institutions are opting for cloud services to support their computing needs.
Here are some benefits you can get through renting GPU in GPU cloud:
- Cost-Effectiveness: Utilizing cloud services reduces initial investment costs, as users can select instance types tailored to their workloads, optimizing costs accordingly.
- Scalability: Cloud services allow users to rapidly scale up or down resources based on demand, crucial for applications that need to process large-scale data or handle high-concurrency requests.
- Ease of Management: Cloud service providers typically handle hardware maintenance, software updates, and security issues, enabling users to focus solely on model development and application.
Rent GPU in Novita AI GPU Instance
Novita AI GPU Instance, a cloud-based solution, stands out as an exemplary service in this domain. This cloud is equipped with high-performance GPUs like NVIDIA A100 SXM and RTX 4090. This is particularly beneficial for PyTorch users who require the additional computational power that GPUs provide without the need to invest in local hardware.
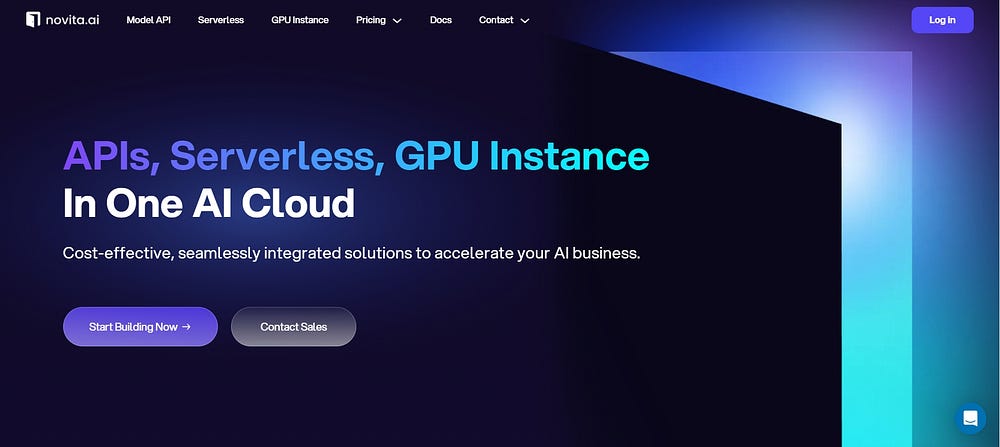
Pros:
- Significant cost savings, up to 50% on cloud costs.
- Free, large-capacity storage (100GB) with no transfer fees.
- Global deployment capabilities for minimal latency.
Cons:
- May require internet access for optimal use.
- Potential learning curve for beginners unfamiliar with cloud-based AI tools.
Take renting NVIDIA A100 for example
As we mentioned above, NVIDIA A100 is one of the best choices for users to optimize the using of AI. And in Novita AI GPU Instance, we also offer NVIDIA A100 80GB, charging based on the time you use.
Benefits you can get:
- Cost-Efficiency:
Users can expect significant cost savings, with the potential to reduce cloud costs by up to 50%. This is particularly beneficial for startups and research institutions with budget constraints.
Now NVIDIA A100 80GB costs about 10,000 dollars in the marketing price. However, by renting it in Novita AI GPU Instance can you save a lot for it charging according to your using time and it cost only $1.8/hr.
2. Instant Deployment:
You can quickly deploy a Pod, which is a containerized environment tailored for AI workloads. This streamlined deployment process ensures developers can start training their models without any significant setup time.
3. Function:
In addition, you can also get the same function as you buy the whole hardware:
- 80GB VRAM
- Total Disk: 6144GB
Conclusion
Choosing the right GPU for AI work is super important because it really affects how well and fast your projects run. The NVIDIA RTX 3090, A100, and RTX A6000 are top picks nowadays due to their awesome performance in deep learning tasks and professional settings. It’s key to get a grip on features such as CUDA Cores, memory capacity, and Tensor Cores if you want to make the most out of AI jobs. With different architectures like Ampere, RDNA, Volta, and Turing around each corner affecting AI results differently; keeping up with what’s new in GPU tech will help keep you ahead in the game of AI research and development. Always be ready to adapt by embracing fresh innovations that can push your AI projects forward towards victory.
Frequently Asked Questions
What Makes a GPU Suitable for AI Rather Than Gaming?
When it comes to a GPU that’s good for AI, what really matters is its ability to handle many tasks at once, support for tensor cores, and having enough memory bandwidth instead of focusing on things important for gaming such as fast clock speeds.
What GPU is best for fast AI?
We recommend you to use an NVIDIA GPU since they are currently the best out there for a few reasons: Currently the fastest. Native Pytorch support for CUDA.
Novita AI, is the All-in-one cloud platform that empowers your AI ambitions. Integrated APIs, serverless, GPU Instance — the cost-effective tools you need. Eliminate infrastructure, start free, and make your AI vision a reality.
Recommended Reading: