3 Best GPUs for AI 2024: Your Ultimate Guide
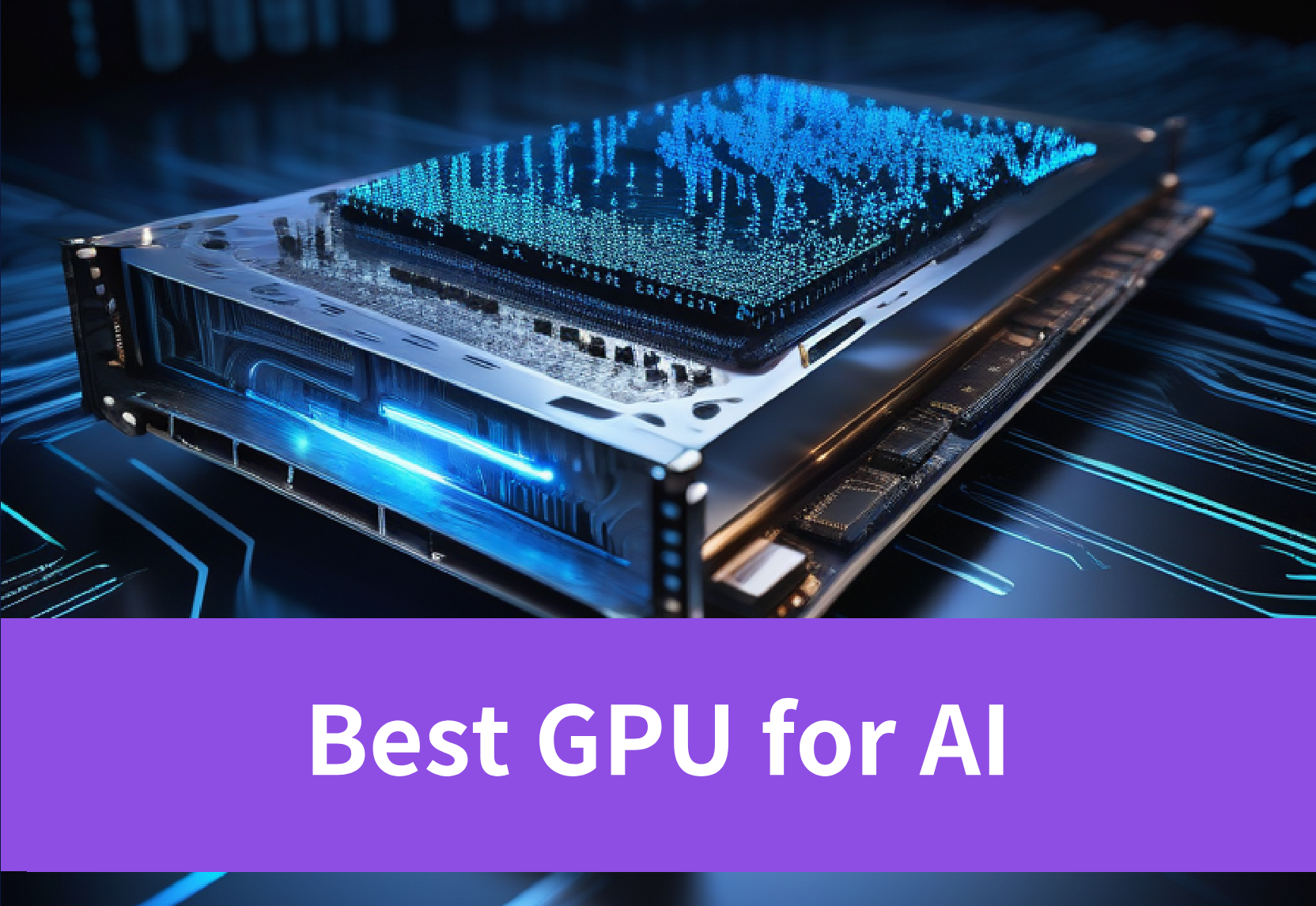
Introduction
AI, or artificial intelligence, has revolutionized various industries like healthcare, finance, and manufacturing by recognizing images and understanding language. GPUs, originally designed for video game graphics, now play a crucial role in powering AI programs. Unlike CPUs, GPUs excel at handling multiple tasks simultaneously, enabling faster learning and decision-making for AIs.
In our deep dive into GPU selection for successful AI and deep learning projects, we’ll explore the top-performing GPUs. Discover what makes them stand out, their speed capabilities, and key differentiators. Whether you’re in data science, researching new technologies, or passionate about artificial intelligence, this guide will emphasize the importance of selecting the right GPU and provide essential criteria for your decision-making process.
Top 3 GPUs for AI in the Current Market
Right now, the market is full of GPUs that are perfect for AI tasks. Let’s talk about three top-notch GPUs that come highly recommended for working on AI projects:
NVIDIA A100: The AI Research Standard
The NVIDIA A100 is a top choice for AI research, thanks to its Ampere architecture and advanced Tensor Core technology. It excels in deep learning tasks and AI training, providing high memory bandwidth and superior processing power. Ideal for deep learning research and large language model development, the A100 meets the demanding needs of modern AI applications.
NVIDIA RTX A6000: Versatility for Professionals
The NVIDIA RTX A6000 is versatile, catering to various AI professional needs. With excellent GPU memory and bandwidth, it handles deep learning, computer vision, and language model projects efficiently. Its Tensor Cores enhance AI acceleration, making it a great choice for demanding AI workloads, balancing high performance with robust handling capabilities.
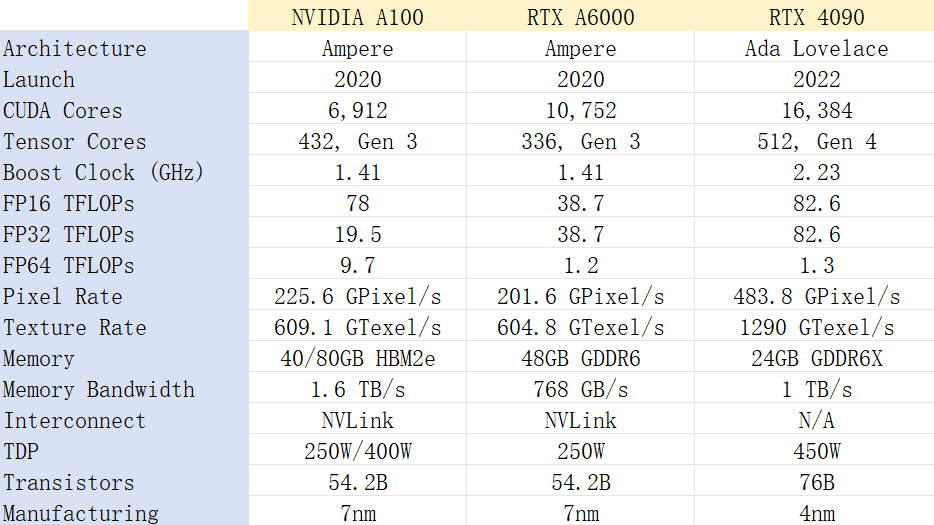
NVIDIA RTX 4090: The Cutting-Edge GPU for AI
The NVIDIA RTX 4090 represents the pinnacle of GPU technology for AI applications. Boasting an unprecedented number of CUDA cores, advanced Tensor Cores, and massive memory bandwidth, it delivers exceptional performance for the most demanding AI tasks. Whether training deep learning models or processing vast datasets, the RTX 4090 ensures unparalleled speed and efficiency, making it the ultimate choice for AI professionals seeking the best in GPU technology.
Key Features to Consider When Choosing a GPU for AI
When picking out a GPU for AI tasks, it’s important to look at several crucial aspects:
Understanding CUDA Cores and Stream Processors
CUDA cores, also known as stream processors, are vital for modern graphics cards, especially in AI tasks. The number of CUDA cores in a GPU affects its speed and power, enabling faster training and smarter AI models. These cores efficiently handle multiple tasks simultaneously, breaking down big computing chores into smaller bits, thus accelerating data processing. When selecting a GPU for AI projects, the number of CUDA cores is crucial for better performance and increased productivity.
Importance of Memory Capacity and Bandwidth
Memory capacity and bandwidth are critical when choosing a GPU for AI tasks. Ample memory allows the GPU to handle large datasets and complex models without running out of space. Faster memory enables quicker data transfer, reducing wait times during calculations, which is particularly beneficial for deep learning projects. For efficient AI model training, a GPU with substantial memory and high-speed bandwidth is essential for smoother and quicker processing.
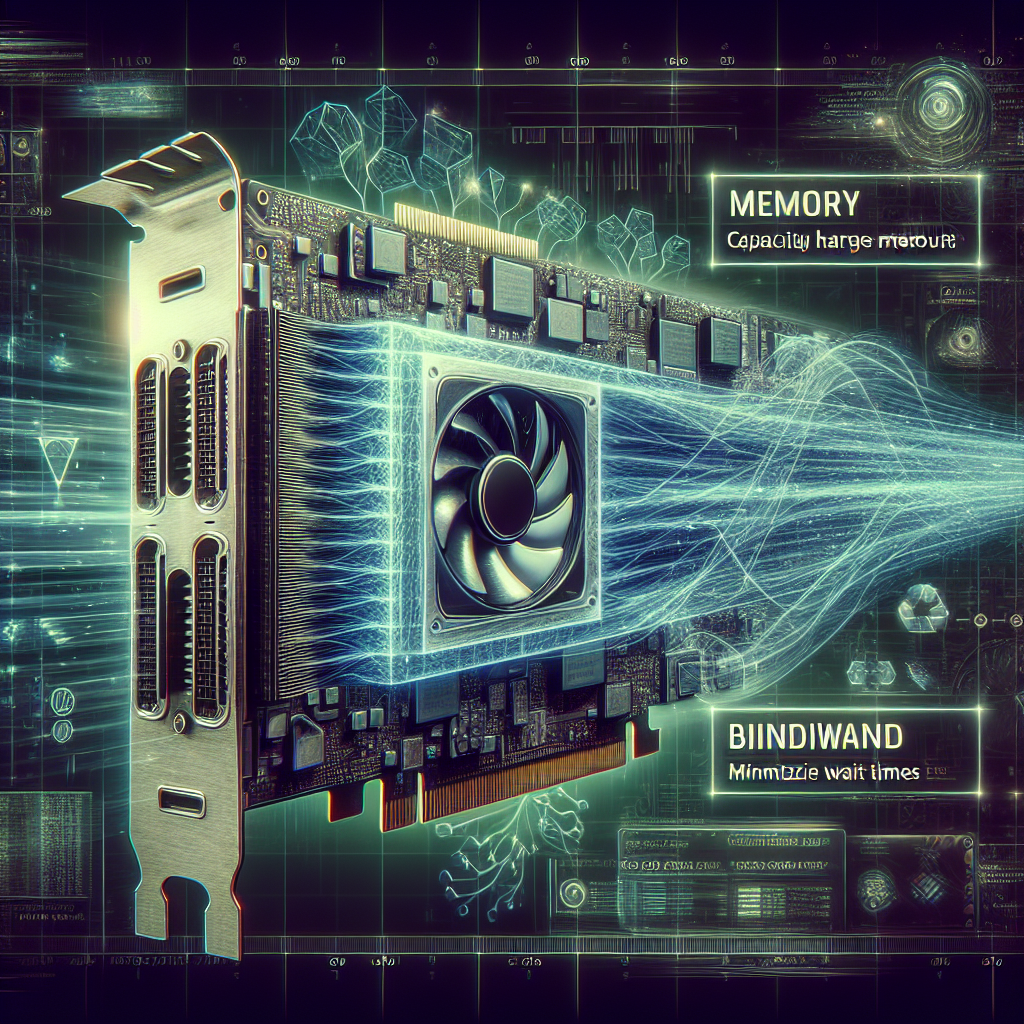
Tensor Cores and Their Role in AI Acceleration
NVIDIA GPUs feature Tensor Cores, specialized for speeding up AI tasks, especially matrix multiplication in deep learning algorithms. Tensor Cores enhance computing power, making training and inference faster by mixing different types of calculations. This efficiency allows for quick processing without excessive memory use or detail loss. For optimal AI performance, selecting a GPU with Tensor Cores ensures faster and smoother operations in machine learning and deep learning projects.
Budget Considerations
When on a budget, finding a GPU that balances performance and cost is key. Look for models that offer a good number of CUDA cores, sufficient memory, and decent bandwidth without the high price tag of top-tier options. Mid-range GPUs often provide excellent performance for many AI tasks without the hefty cost. While they may lack Tensor Cores, they can still handle most machine learning and deep learning tasks effectively, making them a great choice for budget-conscious AI enthusiasts.
Better Way to Get GPU Instead of Buying One
Still worried about the high cost of purchasing a GPU? Here we offer you an alternative choice — try Novita AI GPU Pods!
Novita AI GPU Pods offer a compelling alternative to the substantial capital outlay required for purchasing NVIDIA RTX 4090, RTX 3090, A100 and also A6000 GPU. With Novita AI, users can access cutting-edge GPU technology at a fraction of the cost, with savings of up to 50% on cloud expenses. The flexible, on-demand pricing model starts at just $0.35 per hour, allowing businesses and researchers to pay only for the resources they use. This approach eliminates the need for large upfront investments and ongoing maintenance costs associated with physical hardware. Join the Novita AI Discord to see the latest changes of our service.
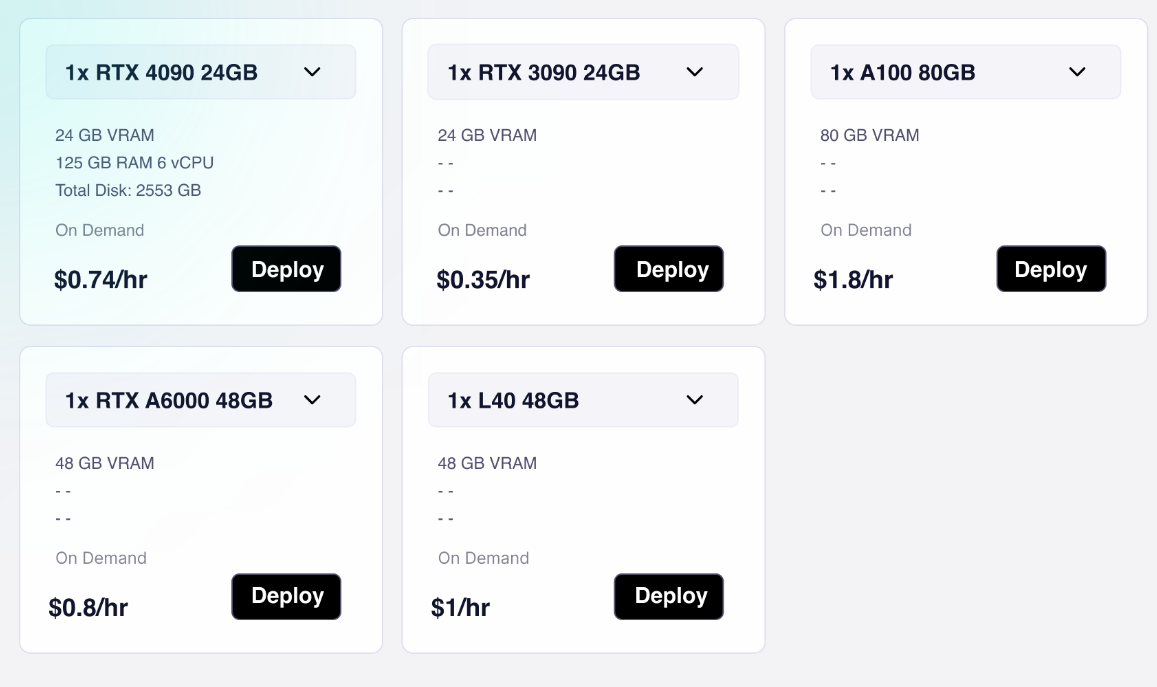
Optimizing Your AI Projects with the Right GPU Configuration
When you’re working on AI projects, it’s really important to think about the GPU setup. You’ve got to look at a few things to make sure everything runs smoothly and efficiently.
Balancing GPU Power with System Requirements
Ensuring your GPU power aligns with system capabilities is crucial for AI projects. Consider the GPU’s power consumption and check if your system supports it. High-power GPUs might need extra cooling or a larger power supply. Balancing GPU strength with system requirements ensures efficient and harmonious operation.
Strategies for Multi-GPU Setups in AI Research
Using multiple GPUs can significantly enhance AI research by speeding up model training and data processing. Connecting GPUs with technologies like NVIDIA’s NVLink improves communication and memory sharing. Optimizing task distribution across GPUs maximizes performance. This multi-GPU approach accelerates AI research and yields faster results for large models.
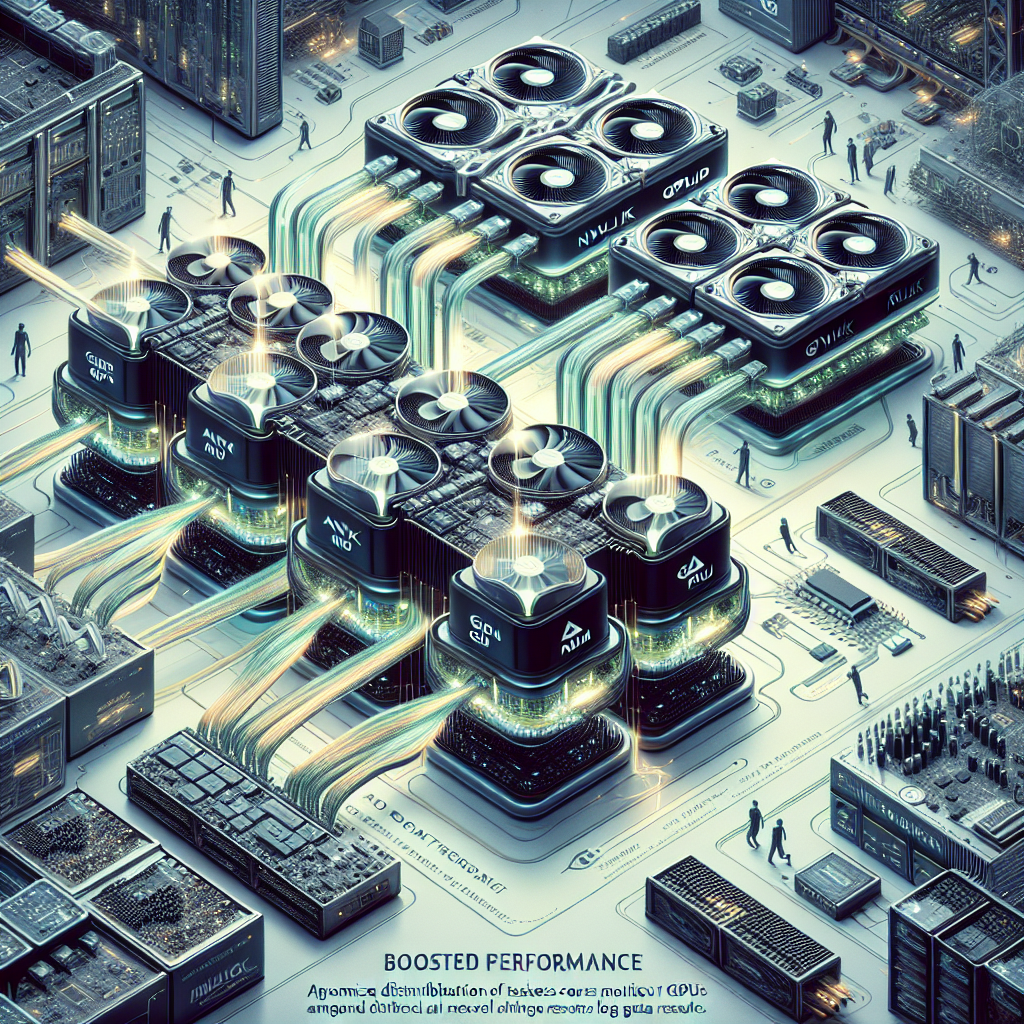
Future Trends in GPU Technology for AI
Looking ahead, the world of GPU tech for AI is pretty thrilling. With machine learning and artificial intelligence getting more advanced by the day, there’s a growing need for even stronger GPUs.
Anticipating the Next Generation of AI GPUs
The future of AI GPUs is highly anticipated as advancements from companies like NVIDIA and AMD promise even more powerful graphics cards. Improvements in memory bandwidth, capacity, and overall performance are crucial for handling large datasets and complex tasks. Staying updated on these developments is essential for excelling in AI research and applications.
Innovations in AI Algorithms and Their Impact on GPU Design
As AI models grow in complexity, GPUs must evolve to provide the necessary power and speed. Enhancements in AI algorithms drive the need for GPUs with faster memory and greater processing capabilities. This synergy between AI advancements and GPU design propels both technologies forward, preparing new GPUs for diverse AI applications.
Conclusion
Choosing the right GPU for AI work is super important because it really affects how well and fast your projects run. The NVIDIA RTX 3090, A100, and RTX A6000 are top picks nowadays due to their awesome performance in deep learning tasks and professional settings. It’s key to get a grip on features such as CUDA Cores, memory capacity, and Tensor Cores if you want to make the most out of AI jobs. With different architectures like Ampere, RDNA, Volta, and Turing around each corner affecting AI results differently; keeping up with what’s new in GPU tech will help keep you ahead in the game of AI research and development. Always be ready to adapt by embracing fresh innovations that can push your AI projects forward towards victory.
Frequently Asked Questions
What Makes a GPU Suitable for AI Rather Than Gaming?
When it comes to a GPU that’s good for AI, what really matters is its ability to handle many tasks at once, support for tensor cores, and having enough memory bandwidth instead of focusing on things important for gaming such as fast clock speeds. With these features in place, the performance of deep learning tasks and overall computational efficiency in AI workloads get a big boost.
Novita AI, the one-stop platform for limitless creativity that gives you access to 100+ APIs. From image generation and language processing to audio enhancement and video manipulation, cheap pay-as-you-go, it frees you from GPU maintenance hassles while building your own products. Try it for free.